Latest Data Science Applications Across 7 Different Industries
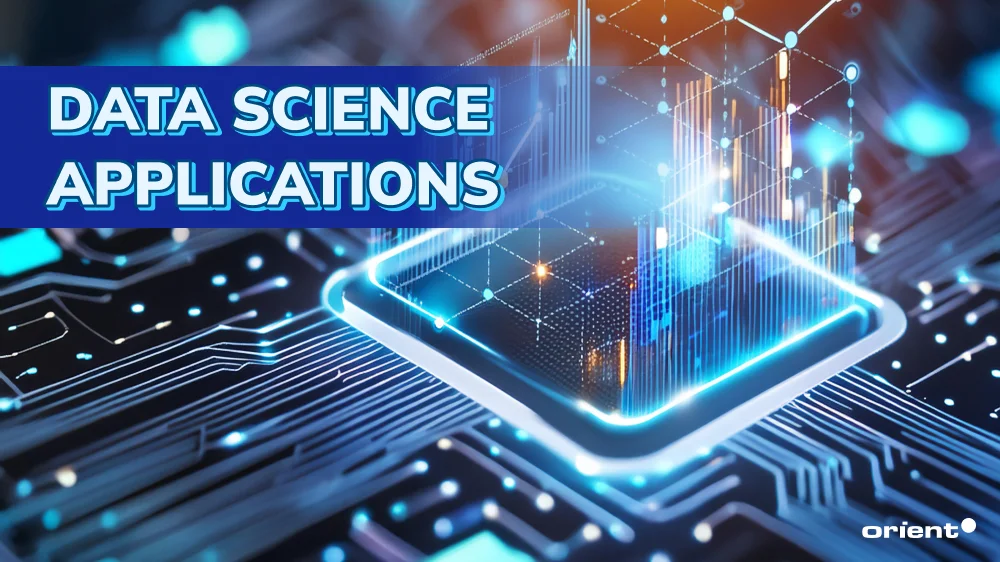
Content Map
More chaptersIt is no coincidence to the success of Coca-Cola today. As we cannot accurately know what the business strategy of this soft drink giant is, many sources reveal that data science is the technology contributing the most to the remarkable growth of Coca-Cola, helping them analyze customer behavior and adjust product lines accordingly.
Data science algorithms, fortunately, are not as difficult to implement as you might think. In fact, many industries have been applying this modern approach to optimize the efficiency of products and processes. In healthcare, data scientists employ predictive analytics to examine historical patient data, thus forecasting future illness occurrences. In finance, investing in machine learning algorithms - a niche of artificial intelligence, helps financial institutions quickly detect anomalies in transactions.
While the implementation of data science methods varies across different sectors, the results of such an investment are always worth it. In which field is your business operating? For more data science applications across industries, let’s take a quick tour of the article below.
Data Science in Healthcare
Virtual Health Assistants
As a profession directly related to human health, healthcare values timely support, even 24/7, from doctors and nurses. While in the past, healthcare facilities were limited to arranging for healthcare professionals to remain on duty overnight to address urgent patient needs, the development of AI-powered chatbots and virtual assistants, which represent popular applications of data science approaches, has partly helped hospitals reduce recruitment costs and the burden of repetitive tasks.
Virtual health assistants with 24/7 availability are capable of providing immediate assistance without the need to wait for office hours or appointments. Although they cannot examine and treat patients like real doctors, AI chatbots can respond to common health-related queries, schedule patient books, or even provide wellness advice based on user input. By integrating conversational AI directly into healthcare facilities’ websites or building a customized one, virtual health assistants improve health management and process efficiency to a new level.
Clinical Trial Recruitment
A clinical trial is a research study conducted to evaluate the safety and effectiveness of new medical treatments and drugs. As it directly relates to human health and the development of medicine, the implementation process of such a trial goes through many different important steps, and clinical trial recruitment is one of them.
No more scanning the vast amounts of data points manually which is time-consuming and inefficient, scientific research centers now promote the role of data analytics to automate the analysis of extensive patient data including genetic information, electronic health records (EHRs), demographic details, etc., and identify suitable candidates to fully meet the complex listed eligibility criteria. Optimizing the clinical trial recruitment process with data science techniques helps reduce the resources needed to enroll participants, contributing to the trial success rates.
Predictive Diagnostics
With data science, patient raw data is not simply information but is also considered a valuable resource housing potential data-driven insights. After being integrated into a unified system and eliminating errors and duplicates, data types such as electronic health records (EHRs), lab results, medical imaging, genetic information, wearable device data, and patient-reported outcomes will be analyzed by machine learning algorithms to identify patterns and correlations that may indicate the early stages of a disease or condition, used for developing appropriate treatment strategies.
This is one of the key applications of data science in healthcare that helps clinicians in making more informed decisions, ultimately improving patient outcomes.
Data Science in Finance
Fraud Detection
Improving fraud detection systems in financial institutions has always been a top concern. Over the past five years, the growth rate for electronic transactions has nearly tripled the overall increase in payments revenue.
While bringing financial activities online offers users the benefit of convenience, this modernization has many potential security gaps as fraud tactics are constantly evolving. Controlling complex user data sets based on traditional methods, which often generate a high number of false positives, is becoming obsolete. Rather than an option, upgrading existing banking systems with modern data science applications becomes a necessity.
Thanks to the use of data science tools like predictive models and data analysis, banks can simplify the process of real-time monitoring and analysis of transactions. Machine learning models with the ability to learn and adapt quickly to new cheating patterns and techniques are effective measures to reduce emerging fraud types. The sooner we detect fraudulent activities, the smaller the potential losses.
Risk Management
Financial risks can arise from any source at any time, causing damage to users if financial institutions lack risk management strategies in place. The truth is that we cannot completely eliminate these threats, but integrating data science into existing operations can contribute to better risk management.
Data science has the capability to continuously analyze and evaluate historical data, market trends, and economic indicators in real time. From unstructured data that seems to bring no value in terms of insight and is often overlooked by humans, data science uncovers surprising aspects that can potentially pose risks of defaults, helping banks improve financial stability while reducing uncertainties.
Data science technologies are even used in stress tests, which are tests that simulate various economic scenarios to predict their impact on the financial institutions. Specifically, Natural Language Processing (NLP) techniques are used to process and analyze all types of data, while machine learning algorithms and statistical models are employed to predict how different variables behave under stress scenarios, thereby evaluating the overall financial health.
Churn Prediction
Once again, predictive analysis comes into play to address issues in financial services, specifically the challenge of maintaining customer satisfaction and reducing unexpected churn rate.
Each customer has different habits of using financial and banking applications, leading to diversity in customer files. That is why financial institutions cannot apply the same customer retention formula to all separate target groups. By letting machine learning algorithms, such as logistic regression and neural networks, do their work—building predictive models to analyze historical data—the data science team better understands what causes a higher customer churn rate, thereby developing personalized strategies like special promotions to improve financial experience accordingly.
Data Science in Retail
In-store Layouts
It is no coincidence that many people assume that selling is state-of-the-art. Did you know that retailers even calculate the placement of goods to suit each customer segment? For example, toys are often placed on low shelves to attract children’s attention. This is a simple but important factor in enhancing the shopping experience and boosting sales.
By applying data science to such processes, retailers take customer satisfaction to a new level. Sensors and cameras, once installed to ensure physical store security, are now used to collect data on customer behavior, including foot traffic patterns, time spent in different areas, and interactions with products. This valuable information is then analyzed to identify patterns such as which areas are most visited and which products are frequently picked up but not purchased, thereby helping shop owners make necessary adjustments to store layouts and optimize product placement.
Augmented Reality (AR) Shopping Experiences
Shopping experiences have evolved to the stage where consumers/customers can even interact indirectly with products through Augmented Reality (AR). However, few people know that data science is the technology behind this evolution in combination with AR.
To create accurate 3D models of products for virtual shopping experiences, retailers first gather data on product dimensions. Data on customer preferences and body measurements are then analyzed to improve the accuracy of virtual try-ons. In the final step, AR applications use real-time rendering, which requires quick processing of large amounts of data to overlay virtual images onto the real world through customers’ smartphones, creating a complete online shopping experience.
Data Science in Marketing
Personalized Marketing
Every customer has different shopping and service needs. That’s why marketing campaigns are often not applied to the entire customer base of a product/service. Instead, retailers focus on segmenting their audience into distinct segments based on behavior, demographics, and preferences with the aim of creating more targeted advertising.
For example, as a tech-savvy person craving new technological advancements, you may come across advertisements for data science training programs on your Facebook page, while your friends’ social networks do not display similar ads.
Data science contributes to almost the entire process, from gathering and analyzing data from various sources such as users’ social media activity, purchase history, and website interactions to segmenting customers into distinct groups based on shared characteristics or behaviors. The results collected at the above stages are the basis for retailers to create tailored content and recommendations that resonate with individual insights.
A/B Testing and Optimization
A/B testing is a cornerstone of modern marketing. Unlike other types of testing, A/B testing requires a large amount of information to determine which variables have the most impact on comparing one-on-one.
Thanks to data science methods, this process is many times faster than traditional ones. This is attributable to the enhanced efficiency of data collection, which also ensures the cleanliness and accuracy of the data, rendering it ready for analysis. Using statistical analysis, marketers can assess whether the differences in performance between the test versions are significant, thereby making necessary adjustments to products and services.
In addition, data scientist teams can use data visualization tools to turn complex A/B test results into graphical representations that are way easier to understand and support decision-making.
Data Science in Logistics
Dynamic Pricing Models
Shipping prices do not always remain the same in every scenario as they can also be affected by a variety of factors such as weather conditions, space availability, shipping lanes, fuel costs, and available capacity. The situation where prices may increase during peak seasons to maximize revenue is an easy example of dynamic pricing.
The lack of timely adjustments in warehousing costs and freight and shipping rates causes businesses to lose many opportunities to attract customers and reduce their competitive advantage. Fortunately, data science tools have come into play and solved many of such problems.
As a technology revolving around big data, data science has the ability to quickly recognize changes in data flow to identify peak times and low-demand periods. Based on the insights generated by the models, prices are then adjusted in real-time to meet market demand and optimize profit margins. This application of data science can also be applied in the retail industry, where product costs may fluctuate depending on competitor pricing, market demand, and available stock capacity.
Real-Time Tracking and Visibility
Rather than operating in a traditional manner, most packages, vehicles, and containers today are attached to IoT devices such as GPS trackers and RFID tags to better monitor the condition and status of the shipment. During the transmission and exchange of data, IoT networks generate huge amounts of real-time data that businesses can extract to generate meaningful insights for optimizing logistics operations.
Unusual signals in data serve as the foundation for businesses to promptly implement necessary changes and improve the overall journey of shipments before they lead to serious consequences. For example, bad weather data helps logistics companies predict potential delays of orders, thereby sending urgent notifications to receivers and maintaining their satisfaction.
Data Science in Education
It is undeniable that teachers have always played a crucial role in developing suitable curricula based on their experiences and observations. However, things are different if they have to manage up to 100 students at the same time, for example. How can they promote the learning efficiency of 100 different individuals?
Data science can help in such a situation. With the ability to analyze unlimited data related to students, data science allows for personalization and efficiency at scale. Unlike humans, who need time to recognize meaningful insights and patterns that may not be immediately apparent, data science has the ability to analyze historical data quickly and make useful recommendations for the teaching process. This technology can be used to predict which students in classes are at risk of dropping out or failing or which teaching methods and materials are most effective, improving the education sector as a whole.
Data Science in Agriculture
Gone are the days when agriculture relied solely on human labor. Technological advancements, including data science, have helped this sector make great strides in recent years. One of the most impactful data science applications in agriculture is precision farming.
By leveraging the connectivity between IoT sensors and information collected from drones and satellite imagery, farmers are constantly updated with crop health, soil conditions, and weather patterns without directly monitoring their crops. The vast volume of data allows farmers to make informed decisions about the optimal use of resources like water, fertilizers, and pesticides in specific areas, reducing waste and improving yields.
With the current rapid pace of modernization, the development of data sets is undeniable. As technology advances and more data becomes available in the future, data science promises to evolve even more strongly in the coming years and reveal many potentials yet to be fully realized. Its capabilities are predicted to not only limit to the above applications and fields but also expand its influence on unexpected niches.
So, instead of hesitating to apply data science to operations, take action today to create competitive advantages that your competitors do not have. Don’t wait to advance your data science career. Contact Orient Software and access our data science services to leverage your business vision.