What is Big Data Analytics? Here is Everything You Need to Know
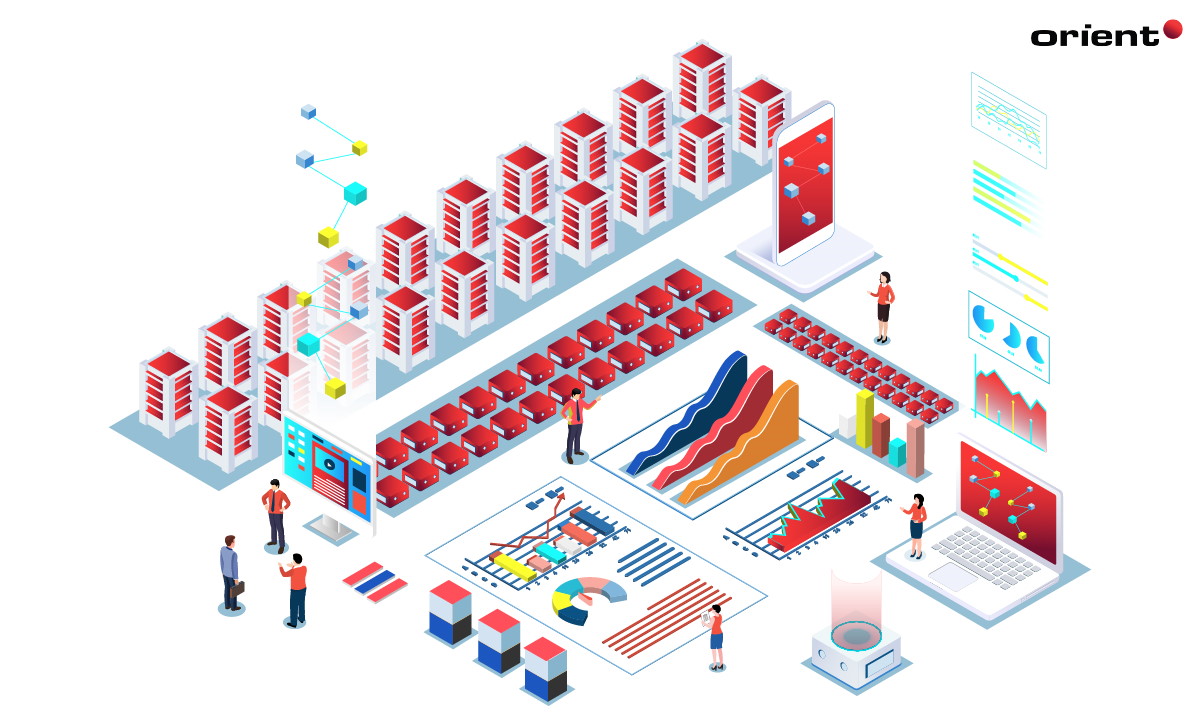
Content Map
More chaptersBig data analytics is the use of tools and technologies to collect, organize, analyze, and present data that is large in volume, velocity, and variety. This kind of data is referred to as ‘big data,’ as it is far too complex to be managed using traditional data analytics methods, such as relational databases. Traditional data analytics is best suited for storing and processing data that is of a similar file format, that comes from a limited number of sources, and that is generated at a pace slower than big data.
Businesses use big data analytics to gather insights previously inaccessible to them, helping them make informed decisions in real time, stay competitive, increase customer satisfaction, and predict future outcomes with greater accuracy.
Understanding Big Data: Sources, Structured and Unstructured Data
With the advent of Artificial Intelligence (AI), mobile devices, Internet of Things (IoT) devices, and social media, the amount of complex data being generated – and the number of places it is coming from – is higher than ever. Thus traditional tools cannot store and process data of this complexity, creating demand for new technology, one that can handle the growing volume, velocity, and variety of complex data sets.
With big data analytics, it is possible to collect, analyze, and present data from a wider variety of sources, and in more file formats, than traditional tools. This could be a temperature reading from an IoT-enabled machine monitoring sensor, social data from Facebook or Twitter to create a personalized digital marketing campaign, or transactional data from an online store to monitor customer behavior and warehouse storage records. Either way, big data analytics helps stakeholders make sense of complex information, identify market trends and hidden patterns, and draw logical conclusions which they can use to make informed decisions.
In addition, big data analytics is capable of processing all types of big data. This includes structured data (data that is clearly identifiable, has a standardized format, and follows a predefined data model), semi-structured data (a subset of structured data that does not follow the standard tabular structure associated with relational databases), and unstructured data (data that is not arranged to a preset data model and therefore cannot be managed in a relational database). This is a huge advantage to businesses, as they can collate data of various types and sources into a single, easy to use platform, allowing them to gain better knowledge of their business operations, their customers, and their strengths and weaknesses.
Types of Big Data Analytics Tools
Big data analytics consists of various tools and technologies that work together to help stakeholders get the most value out of the information that they collect. Each tool assists with different stages of the data analytics process, from data collection and data cleaning to data interpretation and data visualization. Below is a quick breakdown of the most common big data analytics tools in use today.
Cloud computing
Cloud computing is the online delivery of on-demand computing services, such as data storage, servers, databases, software, analytics, and networks. With regard to big data analytics, cloud computing is used to collect, store, analyze, and present big data in a way that is faster, more cost-efficient, and less space-consuming than physical, onsite computing infrastructure. Cloud computing also allows for the remote management of big data; ideal for organizations with hybrid and Work From Home (WFH) arrangements.
Data management
With large amounts of data coming in and out of organizations at all times, systems must be in place to make sure the data is organized, cleaned up, and presented to stakeholders in an easy-to-understand manner. To aid with this task, data management technology establishes repeatable processes for the data analytics system to follow, such as removing duplicate or irrelevant data and formatting structured and unstructured data to make it more readable.
Data mining
Data mining technology scans large volumes of raw data, identifying user and market trends, plus patterns, that may be used to predict future outcomes. The technology can be configured to scan for certain types of data, including specific patterns and trends based on the desired business goals. Data mining software sifts through the noise, adding clarity to the collected data, giving stakeholders a big-picture view of what is really going on.
Machine learning
Machine learning is a subset of Artificial Intelligence (AI). It is used to train machines how to learn, perform complex problem-solving, and accurately interpret the meaning of large amounts of data, including the relationship with each other. With regards to big data analysis, machine learning is used to automatically produce predictive models that can quickly and accurately analyze large swaths of big data, allowing for faster and more informed decision making than traditional tools. By doing so, organizations can discover previously unforeseen opportunities and capitalize on them in a timely manner.
Hadoop
Hadoop is an open-source software framework. It can store and process large amounts of data - including raw and unstructured data - ranging in size from several gigabytes to petabytes of unstructured data (units of information equal to one thousand terabytes). Hadoop can also accept data from a variety of sources, collating it all into a unified user interface. It also takes advantage of distributed processing and storage architecture, using clusters of commodity hardware (as opposed to one large computing system) to spread out the workload evenly, increasing its reliability and scalability.
Big Data Analytics in Action
Big data analytics is used by organizations, small and large, worldwide, to gain meaningful insights into different aspects of their business and make more informed decisions. It is also used to better understand consumer behavior, and then use that information to personalize their experience, creating more desirable outcomes. Here are just a few examples of businesses taking their operations to the next level with big data analytics.
Netflix
As one of the largest streaming entertainment platforms in the world, Netflix prides itself on delivering immediate film and television content to its users, while personalizing the viewing experience with recommendations based on the viewing of past content. To achieve this, Netflix stores billions of datasets in its systems, which include a combination of audiovisual data, consumer metrics, and recommendation engines. With this, Netflix can respond to issues in real time and recommend films and TV shows that closely represent what a viewer is most likely to take an interest in.
Centers for Disease Control
The Centers for Disease Control (CDC) has been using big data analytics for a number of years to improve public health outcomes. In a 1 December 2021 presentation, CDC presenters Juliet Adeola, Allicia Kleine, and Raul Segura-Escano discussed the importance of big data and analytics in their work, including how the technology is critical to shifting the paradigm from historical to predictive data science, enabling a more accurate prediction of public health outcomes. For instance, the CDC recently launched the Center for Forecasting and Outbreak Analytics (CFA), which aims to use infectious disease modeling and analytics to allow for timely, effective decision making when it comes to improving the outbreak and public health response on a local, state, and federal level.
Mint
Mint is a personal finance tool, available to United States and Canada customers, that helps users track their spending and take control of their budget. The service can also break down users’ spending into categories, ranging from groceries and healthcare to entertainment and utility bills, thus giving users a detailed, accurate representation of exactly where their money is going.
To achieve this, Mint uses big data and analytics to collect all sorts of financial data from their users, analyze data, and then present it to users in a way that they can understand easily. It can present insights into a user’s shopping habits, favorite products and services, the time of day when spending is most likely to occur, and much more. The service then uses this information to help their users better manage their spending habits, as well as take advantage of exclusive discounts through Mint on select retailers and events.
Enjoying the Benefits of Big Data Analytics for the Future
For companies and organizations that are already using big data analytics, they are quickly realizing the benefits of this service. This is aided by the fact that the global Big Data and Analytics market was valued at 240 billion U.S. dollars in 2021, according to independent research by Statista.
In addition to this, 27 percent of business executives say that their big data initiatives are profitable, while 45 percent say that they consistently break even, according to a survey of 210 executives by Capgemini. However, as promising as these figures are, research also shows that 43 percent of IT decision makers are concerned that their IT infrastructure won’t be able to meet future demand, based on a 2020 report by Dell Technologies.
With findings like this, it is clear that businesses around the world are interested in harnessing the true potential of big data and analytics, with those that are seeing promising results, while also dealing with concerns of scalability as the amount of data generated on a daily basis will increase over time. For this reason, organizations wanting to take advantage of big data analytics – or improve their existing data analytics systems – are encouraged to do so, if they are to be prepared for the future.