
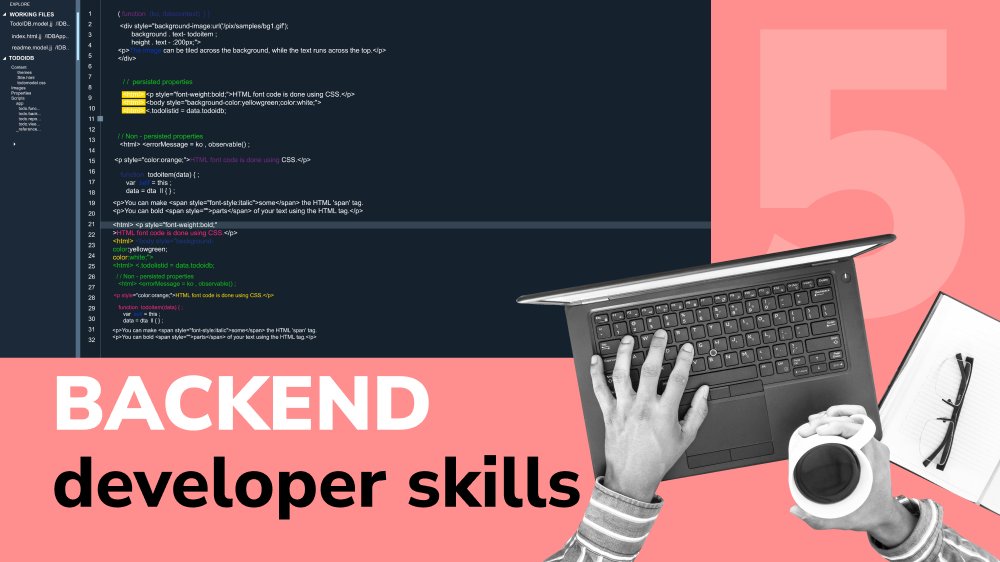
5 Backend Developer Skills You Need to Learn Today
Want to build the brains behind the web? Discover the 5 essential backend developer skills and launch your developer career!
Do you ever feel like you’re surrounded by an ocean of information but thirsty for actionable insights? You’re not alone. In today’s digital age, businesses are generating data at an unprecedented rate – Forbes estimates that 2.5 quintillion bytes of data are created daily. That’s a staggering number, and while data holds immense potential, it can quickly become overwhelming.
The good news? You don’t have to become a data scientist to unlock its power. Data optimization – the process of transforming raw data into clear, actionable insights – can be your life raft. This article will equip you with the knowledge and strategies you need to breathe new life into your data and finally turn information overload into informed decision-making.
Key Takeaways:
Data optimization is the process of improving the performance and efficiency of data management systems by reducing data redundancy, maximizing data quality, and optimizing data storage and retrieval. It involves analyzing the current data infrastructure and identifying areas where improvements can be made to increase the speed and accuracy of data processing.
The purpose of data optimization is to address challenges associated with poor data quality, such as inconsistent formats, missing values, duplication, and inaccuracies. Optimized data can provide numerous benefits for organizations, including improved data accuracy, faster data processing times, reduced storage costs, and increased productivity. By ensuring accurate and reliable data, organizations can make more informed decisions, gain deeper insights, and drive better business outcomes.
The data optimization process can be applied to various types of data, including structured data, semi-structured data, and unstructured data. Structured data, such as data stored in databases, can be optimized through techniques such as data normalization, while semi-structured data, such as data stored in XML or JSON files, can be optimized through techniques such as data compression and data deduplication. Unstructured data, such as data stored in text files or multimedia files, can be optimized through techniques such as data compression, data archiving, and data indexing.
Data optimization encompasses a variety of techniques used to transform raw data into a valuable asset. Here are some of the most common data optimization techniques:
Data cleansing, also known as data scrubbing or data cleaning, is a critical process in data optimization. It involves identifying and correcting errors, inconsistencies, and inaccuracies within datasets. This process is essential to optimize data for accurate analysis and decision-making.
Sophisticated data quality tools and techniques are available for data cleansing processes. Some common techniques include:
Data cleansing tools such as OpenRefine, Trifacta, and Talend offer automated capabilities to streamline and expedite the data cleansing process.
Data deduplication is the process of identifying and eliminating duplicate data entries within a dataset. Duplicate data can arise from various sources, such as data integration processes, data migrations, or human error during data entry. Data deduplication is significant because it improves data quality, reduces storage requirements, and enhances data analysis accuracy.
There are several methods for identifying and eliminating duplicate data:
Data deduplication can be performed manually or with the help of automated tools and algorithms, such as Apache Spark’s deduplication functions or specialized data integration software.
Data compression techniques are employed to reduce the size of data while preserving its integrity and usability. Data compression is vital for optimizing storage capacity, improving data transfer efficiency, and minimizing network bandwidth requirements.
There are primarily two categories of data compression methods:
When applying data compression, it is essential to strike a balance between reducing data size and maintaining data integrity. While compression can significantly reduce storage requirements, overly aggressive compression algorithms or excessive data loss in lossy compression can compromise the fidelity and accuracy of the data.
Data aggregation involves combining multiple data points or records into a single representation. It helps summarize and condense data, reducing the overall volume and complexity.
Some common aggregation methods include:
When performing data aggregation, it is crucial to consider the granularity of the aggregated data and the level of detail required for analysis. Aggregating data at too high a level may result in the loss of important information, while aggregating at too granular a level may lead to excessive data volume and complexity.
Having explored the essential tools and techniques, let’s delve into some key strategies that can be implemented to optimize your data and maximize the insight values:
Prioritizing relevant data is a key strategy in data optimization efforts. This involves identifying and focusing on the most valuable data that can drive actionable insights. Not all data is equally important, and focusing on irrelevant data can lead to wasted resources and missed opportunities.
There are various techniques for defining data relevance and importance. These include data profiling (to understand the characteristics of the data), correlation analysis (to identify relationships between variables), and business impact analysis (to assess the potential impact of the data on business outcomes).
Implementing data governance is another crucial strategy for data optimization. Data governance plays a significant role in enhancing data quality and ensuring that the data is reliable, consistent, and usable.
Data governance involves establishing policies, standards, and processes for data management. This includes defining data ownership, implementing data quality controls, and ensuring data privacy and security. Tools like IBM InfoSphere, Informatica Axon, and Collibra can assist in implementing effective data governance.
Automated big data analytics and AI-empowered platforms are at the heart of modern data optimization. These platforms handle large volumes of data, including infrequently accessed data, and provide capabilities for data storage, processing, and analysis. They enable data visualization, which helps in understanding complex data patterns and trends.
There are numerous use cases and benefits of utilizing AI-driven data optimization tools. For instance, machine learning algorithms can be used to automate the process of data cleansing and deduplication. They can also be used for predictive modeling, anomaly detection, and data visualization, thereby enhancing the value derived from the data. Tools like RapidMiner, DataRobot, and H2O.ai provide robust capabilities for AI-driven data optimization.
In conclusion, data optimization is not just a process but a strategic approach that can significantly enhance business efficiency and decision-making. By prioritizing relevant data, implementing robust data governance, and leveraging the power of automation and machine learning, businesses can transform their raw data into meaningful and actionable insights.
Embracing data optimization can lead to improved operational efficiency, more accurate business forecasting, and, ultimately, a competitive edge in today’s data-driven marketplace. However, the journey to effective data optimization requires expertise and a deep understanding of data science.
This is where Orient Software comes into play. As a reliable outsourcing company with a team of experts in data science, Orient Software can help you develop and implement your data optimization project. With our expertise, you can navigate the complexities of data optimization and harness the full potential of your data.
So, take the next step in your data journey. Consider Orient Software for your data optimization needs and unlock the true value of your data. Remember, in the world of business, data is the new gold, and optimizing it is the key to uncovering its true value.
Want to build the brains behind the web? Discover the 5 essential backend developer skills and launch your developer career!
Finding the right client management software does more than simply track your customer's movements. It is the tool you must have to boost sales revenue.
Low-code platforms are a quick and simple way to build small-scale apps. But they do have some caveats. Here is everything that you need to know about them.
Every developer needs to have a comprehensive list of front-end development tools to excel in their job.
Learn about the different types of APIs, how they work, what you use them for, and how to choose the best APIs for your project. Contact Orient Software today!