
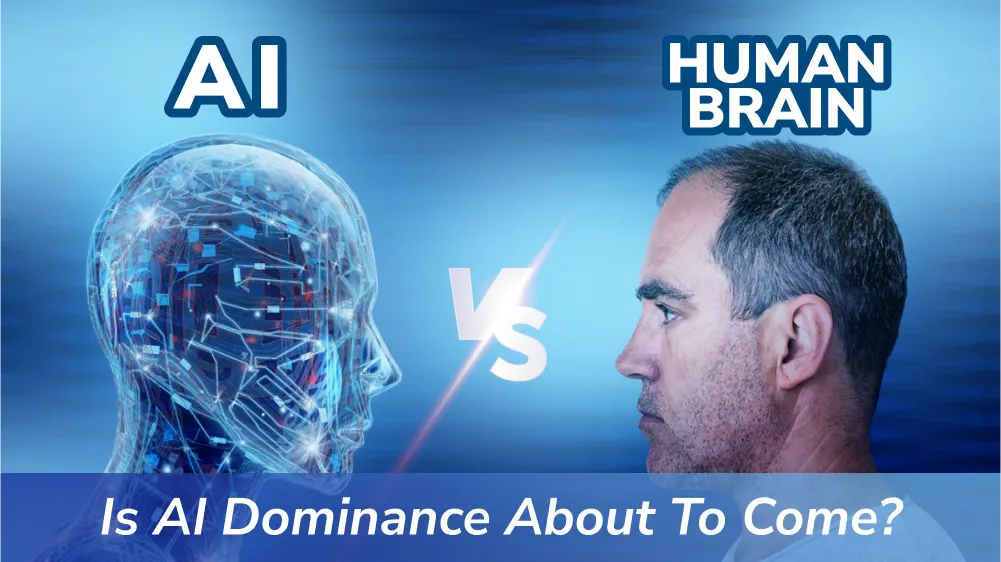
AI vs. Human Brain: Is AI Dominance About to Come?
The debate between AI vs. human brains has long been an endless topic in society. Human intelligence currently surpasses AI, but will it last?
So, Artificial Intelligence. AI is definitely not some new knowledge, especially to business leaders; however, 85% of AI projects fail to successfully deliver the correct results, terrifying new leaders from implementing the never-stop-trending technology. Nevertheless, this should never stop you from exploring and taking advantage of AI technology. Why? Simply put, because your competitors are.
Whether to build your own AI apps or purchase AI services like chatbots, predictive analytics, business forecasting, or natural language processing from third parties, you should be fully ready before making the first step. So how can you start your first AI project? Are there big concerns that you need to watch out for?
Let’s go through the bigs and smalls of AI in order to get the clearest view and a deeper understanding of AI and its many benefits while solving the most common AI issues for all AI projects.
Artificial Intelligence or shortly AI is intelligence demonstrated by machines, as opposed to human intelligence or natural intelligence displayed by animals. AI is a wide-ranging branch of computer science concerned with developing intelligence in machines and building smart machines capable of delivering and performing tasks that are typically done and performed by humans.
There are two primary AI types, which are Artificial General Intelligence (AGI), aka Strong AI, and Artificial Narrow Intelligence (ANI), aka Weak or Narrow AI with AGI that is capable of performing real intelligence similar to that of humans, while ANI has to be programmed to do so. Currently, only ANI is developed, and there is an ongoing debate regarding whether AGI is possible, or at least possible in the near future.
Other subfields of AI that are commonly talked about are Machine Learning and Deep Learning, with Machine Learning as a field of study devoted to building machines that can learn from data and continuously adapt and improve themselves, while deep learning works with layers of artificial neural networks to imitate how the human brain works.
Before going deep into how to start your first AI project, it is vital to know the different types of AI development styles and figure out which is the most suitable for your business.
Large tech enterprises such as Microsoft, Amazon, IBM, or Google all offer Artificial Intelligence as a Service (AIaaS) to all companies and individuals interested in AI but do not have the budget or the manpower to develop their own AI applications. These services range from small tasks such as chatbot or personal assistant to complicated functions such as predictive analytics or deploying ML projects.
The biggest advantage when it comes to these services is that you are guaranteed a working service for your budget, with high-tech security and maintenance. There is a fixed fee, usually weekly, monthly, or annually, and outside of that, you are stress-free. You also have no worry about updating your infrastructure and adapting to new tech, as these services generally keep up with the newest, most advanced technologies.
Since some businesses rely on AI to run general assignments, AIaaS provides fast and cost-effective solutions with minimal effort. If developing an in-house AI team is out of the equation, looking for a reliable third party to provide your company with a trustworthy AIaaS would be your safest bet.
Fast and cost-effective
AIaas is your AI pay-as-you-use option where you can instantly start using your product without going through a long and time-consuming development process, at a fraction of the cost, and without worrying about testing or maintenance. Your applications are also secured by top tech companies and are up to date with all the latest technologies.
Flexibility
It is easy to scale up and down as all you need to do is change your subscription option if you want more storage or users on your application. You are paying for what you are using for your business at any point in time, without those extra costs. Also, you don’t need to hire more employees or reduce headcount once development starts and finishes.
Perfect for smaller scale projects or businesses
As budget and time are the most concerning issues for smaller businesses, AIaaS is the safest bet for your business’ AI solutions. There are quite a lot of available options to choose from, which makes sure that you can get the best AI solution for your needs, especially if your business does not rely heavily on the use of AI.
Reliance
You are paying for a service at the end of the day. This means, most likely, that the application is not fully customized to fit your personal needs. If any issue arises, even if the third party has a great support team, your issue might not be solved immediately or prioritized over other people’s issues. The bigger the tech company is, the longer the customer support queue - this is a given fact.
Questionable Security
All your data is shared with your chosen third party, and if you are renting service from a less reputable third party, you might have some concerns over your data security. Even Facebook, one of the world’s biggest tech giants, is known for security issues and using your personal data for their own gains.
Long-term Costs
How long are you going to rely on AIaaS? In 10 years, maybe the fees you are paying will be higher than how much it would cost if you develop your own AI application. Maybe the time waiting for technical support affects your company’s revenue, or if there is maintenance that cuts your AI services right at a vital time. Imagine losing your services while striking a crucial deal, and even if not, it can cause insane loss - such as when Google suffered a 5-minute power loss and experienced a loss of $545,000 in revenue - not including losses from other businesses which were using their services.
Data Limit
Even though there are many options for AIaaS subscriptions, if your company needs a large infrastructure to store all your data or there is a lot of processing to do, the combined cost of all services might add up to an enormous amount, especially in the long run. Some AI companies only offer storage for up to a certain amount, and most of your data is stored in the cloud instead of your own server, resulting in delay, lag, or might prohibit you from fully taking advantage of AI and its full potential.
The other option besides paying for a third party’s AI services is to build your own AI team or AI department. This is generally considered the better option long term as, eventually, all businesses will rely more and more on AI, as this technology is powering most business procedures, taking over old-fashion technologies and processes. Even though the up-front costs might be immense, the end result can outweigh the cost. Especially, there are several reasons that make building an internal AI team not just an optional choice, but a must, such as:
Better Customization
When your business relies heavily on AI and its functions, you might need an AI application that is tailored to specific needs and demands. This is especially true when complicated customization is needed, and you need to modify the application frequently.
Ownership
Having an in-house team provides ownership for all your AI applications - they are your company’s assets and intellectual properties. There will be no worry about leaking your company’s secrets to other third parties or fighting over the rights to ownership. You can also rent out your AI application for extra revenue if you choose to.
Independence
No more waiting for customer support, no longer praying for better updates, having an in-house AI team makes sure that all your needs are prioritized at any time of the day, and all features are personalized to your demands. Changes no longer pose an issue, and you have full control over what your application can do and how it should run.
Long-term Cost
If your company requires complicated AI solutions for its core functions, chances are, you need an in-house AI team. Not only does it prevent data leakage, but an in-house AI team also makes sure that all your needs are responded to in a timely manner and makes your revenue and benefits the top concern. In the long term, it might cost less than paying for an AIaaS, or hiring an offshore AI team, considering the faster turn-around time and time to market, as well as how the solutions are adapted to your business only.
Cost
To have an AI team, you need at least a few top-paying experts to run the team, as well as plan and develop solutions strategically. In addition, the cost of running a high-tech team can add up to a huge sum. Since tech is a very competitive field for recruiting talent, you are competing with thousands of other companies for true experts and specialists, and the best option to gather talent is to provide the best package that is attractive enough - which, again, means extra costs.
Lack of Talent
As the IT market is increasingly competitive, the race to attract outstanding talent is getting harder and harder to win. Not only do you have to have an inviting salary and benefits, but you have to also possess a great working environment and provide a solid career path for your employees in order to retain them against your competitors.
Increased Commitment
The biggest obstacle to having an in-house AI team is the dedication and commitment to building a team. You have to hire from A to Z, from the person in charge to the people working on every aspect of the project. This is not a one-day journey, and your company might need to devote a lot more time and budget than expected.
Another somehow “best of both worlds” solution when you want a mixture of both AIaaS and an in-house AI team is to outsource your AI project. This means you are leveraging a third-party IT company to develop and maintain your AI application. This is considered a perfect solution for businesses that need customized, personalized AI solutions, yet might not have the time, budget, and qualified talent to carry out the results by themselves. Hence, they need the support of high-quality AI experts to develop their AI applications for them.
Scalability
As the outsourcing company handles all your staffing needs, you do not have to worry about scaling up and down, hiring new talent, or firing current employees for your project. We all understand the pain of letting go of your precious employees because their work is done, and by outsourcing your project, you can be at ease that the employees can be transferred onto another project that needs their help instead of being left without a job.
Time and Cost Optimization
Even though outsourcing might not be as fast as paying for AIaaS since you are still developing software, it is indeed much faster than starting your in-house team. You have no worry about hiring a Head of the IT Department or whether the experts are qualified and have the skills they need - your outsourcing partner handles all of those for you, and chances are, they have the right people ready for all your needs.
Larger Talent Pool
Since you are offshoring your project elsewhere, you are tapping into a whole different talent pool with unlimited potential and choices. Since the IT market is increasingly competitive, the demand for IT talent is at an all-time high. Outsourcing is an opportunity for you to find IT experts that can fulfill all your requirements elsewhere without going through the extra length of recruiting and training.
Dependence
You are relying on a third party to develop your perfect product, which is a good and a bad thing. First, this means you can concentrate on your business’s strong points and leave the technical aspect to your IT partner. However, that also means you have less control over your AI product. Are they going to deliver the right product for your needs? Or better yet, are they going to deliver?
Quality
As another company is handling your project, quality might be a big concern. This is true, especially when you are collaborating with your outsourcing partner for the first time. Make sure to check all their former partners and case studies before signing the contract, so your partnership and delivery happen smoothly.
Barriers
If you are working with an offshore partner, chances are they are not sharing the same language and culture as your company. This can become a challenge in the long run, especially if sharing your ideas and vision becomes an obstacle. It’s recommended that you visit your partner’s office and talk to your offshore team before starting the project to be guaranteed that your offshore team can understand all your expectations and requirements appropriately.
Artificial intelligence development is not something that you are able to rush and succeed; on the contrary, most such AI projects end up failing. According to a recent survey from Databricks, among 90% of companies working on AI development projects, only one in three is considered successful. Therefore, take every step of the way carefully if you would like to set your AI projects up for success. What steps? Here are five steps you should take respectively to embark on AI development.
First and foremost, question yourself about the ultimate goal as well as the value you wish to achieve from your AI project. Would you like to simply build a basic solution with artificial intelligence or create a complete AI-driven tool that serves your business goal? What benefits does your company hope to bring out by investing in such technology? How will this affect or change your business objectives?
Ask yourself all the questions that come up in your mind about the core value you wish to reap from artificial intelligence technology, try to analyze your AI initiatives from different angles and be clear about the final objectives of your AI projects. Once the management and executives have a basic understanding of the core value and objectives of what they are about to do, they will have a clearer picture of what they should do next and how much they should invest in this.
This step sounds theoretical, isn’t it? But a majority of businesses that are initiating AI projects have no idea of what they are expecting to achieve or where the project will head, eventually ending up failing or wasting time and resources for nothing. They simply chase after artificial intelligence development as everyone else is doing it or because they would like to make their businesses appear as if it is up-to-date with the latest technology trends. This driver is known as FOMO (fear of missing out), and it is avoidable with a little more critical business thinking. So, do not follow in their footsteps if you wish your AI project to come to fruition.
Instead, try to make clear the objectives of your AI project from the very beginning. Remember to identify the pain points of your business, and AI solutions should be born to resolve them for you. Moreover, the goal you set should be realistic. Of course, you can dream high with your project, but in the end, the anticipated outcome must be feasible in the context of your business. All of these things must be well-documented before mapping out a project plan.
There is a wide array of technologies and software solutions available in the market that you can make use of for your AI project, ranging from the programming language for AI, development tools, and testing tools to cloud services as well as big data solutions. For example, if you are planning to build a computer vision application, you will need to consider TensorFlow, Keras, Caffe, Torch, and so on, while in natural language processing, common choices include NLTK, spaCy, Gensim, and others. Therefore, it can be really overwhelming for businesses to make the decision on which tech stack is the most suitable for their project.
The key here is first to understand your project requirements and then match them with the right tools and technologies. Consider what your project is trying to achieve, as we emphasized previously and what data you have available; only then can you start narrowing down your options and select the good resources for your project.
Two recent reports by Gartner revealed that the percentage of AI and machine learning projects that fail to deliver accounts for 85%, while only 53% of projects make their way from prototypes to production. Unfortunately, the key to the success or otherwise failure of AI projects lies within the people factor more than the technology itself. Therefore, the next step to getting started with AI is to have the right talent by your side to run and manage your AI project. Or it means building an AI development team with the necessary skills and experience that are required to see your project through till the end.
Similar to how to hire software developers, you need to consider a lot of factors before you can hand-pick the right talent for your team. Select those who have actual experience in developing AI applications or at least have a good understanding of machine learning concepts. You can start by finding data scientists or data engineers with a background in mathematics, statistics, and algorithms, as they will be able to apply their knowledge to understand and solve various issues related to data. Make sure you also have people who are good at critical thinking and problem-solving so that they can find creative solutions to difficult challenges.
You can always start by looking internally first, see if there are any employees with the relevant skillsets and experience, and then move on from there. Alternatively, outsourcing a dedicated development team or hiring IT team augmentation services is a better choice in case you have no or do not have enough in-house resources available for the upcoming AI project. Developing AI talent within your organization or hunting them is such a daunting task. Instead of struggling with the recruiting, training, and management processes, you can enjoy having experts working for you on an as-needed basis. Not to mention, this will also save you time and money in the long run. But whatever route you choose to take, make sure that you do not forget the importance of having the right talent on your side, as they will be the ones spearheading your initiatives.
Data is the core of any AI project, as it is used to train machine learning models so that their algorithms can learn how to make predictions. For this reason, you will not be able to train your machine learning models or get any valuable insights from data if you do not have enough high-quality data to work with. In other words, your data should be vetted to ensure it is clean, well-labeled, and accurate for you to get the most out of it. Accordingly, it is significant to prepare and optimize data before feeding it into the machine learning models.
According to the AI Creation Hierarchy Of Needs, the data normally goes through 5 main stages before making its way to artificial intelligence or deep learning development: Collect, move/store, explore/transform, aggregate/label, and learn/optimize. But we can simply wrap them all up into two main phases – data preparation and optimization – that cover all the stages above:
Once you have optimized your data and got it in the ready position, you will be one step closer to developing high-quality machine learning models. What’s next?
No skyscraper stands tall without a solid foundation, and neither does an AI project. Now that you get the two most fundamental things – people and data - in place, it is time to roll up your sleeves and get started with AI by building its foundation. Normally, projects involving AI-driven technologies can be a longer venture than we think, so any business seeking the power of AI should approach it as something that can be scalable and long-lasting over time. Unlike any basic application, you complete and launch it, and done.
Whether it is supervised or unsupervised learning, there is always a need for a system that can be continually optimized as new data comes in. This means the AI model needs to be nurtured continuously. Artificial intelligence development is no small feat since the AI model needs to be nurtured continuously. If not, it soon ends up being outdated and no longer utile. The data scientists have to constantly conduct ML experiments and retrain the models to not only make good predictions but also refine the models over time as the data varies and evolves.
In addition to the five-step guide outlined above, there are a few things you should take time to consider before embarking on the AI development venture:
But perhaps our eyes seem to be clouded by the overrated power of artificial intelligence so that we almost forget the nature of an AI project is still an IT project, and it should not be treated differently. All of the management processes, ranging from ideation and requirements gathering to development, testing, and deployment, that are carried out around an IT project should be leveraged for AI as well. The best way to get started with AI is to integrate it into your existing IT infrastructure and processes. You will be able to avoid potential disruptions and ensure a smooth transition for your team.
Training machine learning models can be computationally intensive and requires a lot of resources. Not to mention, deploying ML models in production can also be challenging. In order to overcome these challenges, you need to invest in IT infrastructures, such as servers, storage, and networking. By preparing the right IT infrastructure in advance, you will be able to train machine learning models quickly and deploy them at scale. Businesses that familiarize themselves with advanced digital technologies, such as cloud computing, mobile, and web application development, big data analytics, etc., are more likely to get started with AI projects easier than those that don’t.
AI projects tend to be complex and often take longer than expected to finish. As a result, it is important to adopt a lean and agile approach in order to manage them effectively. How? Start small by developing a proof of concept (POC) or a minimum viable product (MVP). By doing so, you will be able to validate your ideas quickly and get feedback from users early on. Iterate quickly and effortlessly based on the feedback you receive and continuously improve your AI project. In addition, it is significant to reduce risks and avoid stagnancy issues incurring within the development lifecycle. Accordingly, you should structure your project in the first place with an estimated timeline and concrete milestones and stick to them.
The debate between AI vs. human brains has long been an endless topic in society. Human intelligence currently surpasses AI, but will it last?
Are you seeking the ideal programming language for your AI project? Explore this curated list of the top ten languages and their corresponding frameworks.
If you want to know how to become an AI prompt engineer, know that you need more than technical expertise to succeed in this emerging field.
The race to dominate AI space and be the first to develop breakthrough AI technology presents companies with many challenges, which are outlined in this.
Find out what AI code generation is, the best tools, and what to consider when choosing a software development team in a post-AI-driven world.